Jun 20, 2024 |
Another year, another update: I have published two blog posts on the ICLR 2024 blog post trackâwhile working at Midjourney. Iâm grateful for the opportunity to work on this open research on the side.
In particular, one of the blog posts was selected as a Highlight of the blog post track:
Both blog posts are available on the ICLR 2024 Blogpost Track website.
|
Dec 1, 2023 |
Another year, another set of papers. This year was dominated by writing up my thesis and defending it. Iâm also very happy to have joined MidJourney as a Research Scientist at the end of September. Thus, this year was mostly about wrapping up some loose ends into papers:
- âDoes âDeep Learning on a Data Dietâ reproduce? Overall yes, but GraNd at Initialization does notâ (Kirsch, 2023)
- âBlack-Box Batch Active Learning for Regressionâ (Kirsch, 2023)
- âStochastic Batch Acquisition: A Simple Baseline for Deep Active Learningâ (Kirsch et al., 2023)
And finally my thesis: âAdvancing Deep Active Learning & Data Subset Selection: Unifying Principles with Information-Theory Intuitionsâ (Kirsch, 2023)
|
Dec 1, 2022 |
Very happy to have published a few papers at TMLR (and co-authored one presented at ICML) this year and to have (joint) co-authored papers that we will present at CVPR and AISTATS next year:
- âPrioritized Training on Points that are Learnable, Worth Learning, and not yet Learntâ, ICML 2022 (Mindermann* et al., 2022)
- âA Note onâAssessing Generalization of {SGD} via Disagreement"", TMLR(Kirsch & Gal, 2022)
- âUnifying Approaches in Active Learning and Active Sampling via Fisher Information and Information-Theoretic Quantitiesâ, TMLR (Kirsch & Gal, 2022)
- âDeterministic Neural Networks with Appropriate Inductive Biases Capture Epistemic and Aleatoric Uncertaintyâ, CVPR 2023 (Highlight) (Mukhoti* et al., 2023)
- âPrediction-Oriented Bayesian Active Learningâ, AISTATS 2024 (Bickford Smith* et al., 2023)
Several of these can be traced to workshop papers, which we were able to expand and polish into full papers.
|
Jul 24, 2021 |
Seven workshop papers at ICML 2021 (out of which five are first author submissions):
Two papers and posters at the Uncertainty & Robustness in Deep Learning workshop:
Four papers (posters, one spotlight) at the SubSetML: Subset Selection in Machine Learning: From Theory to Practice workshop:
Active Learning under Pool Set Distribution Shift and Noisy Data
Andreas Kirsch, Tom Rainforth, Yarin Gal
SPOTLIGHT
(also accepted at the Human in the Loop Learning (HILL) workshop)

or as Poster PDF version as download.
A Simple Baseline for Batch Active Learning with Stochastic Acquisition Functions
Andreas Kirsch, Sebastian Farquhar, Yarin Gal
(also accepted at the Human in the Loop Learning (HILL) workshop)
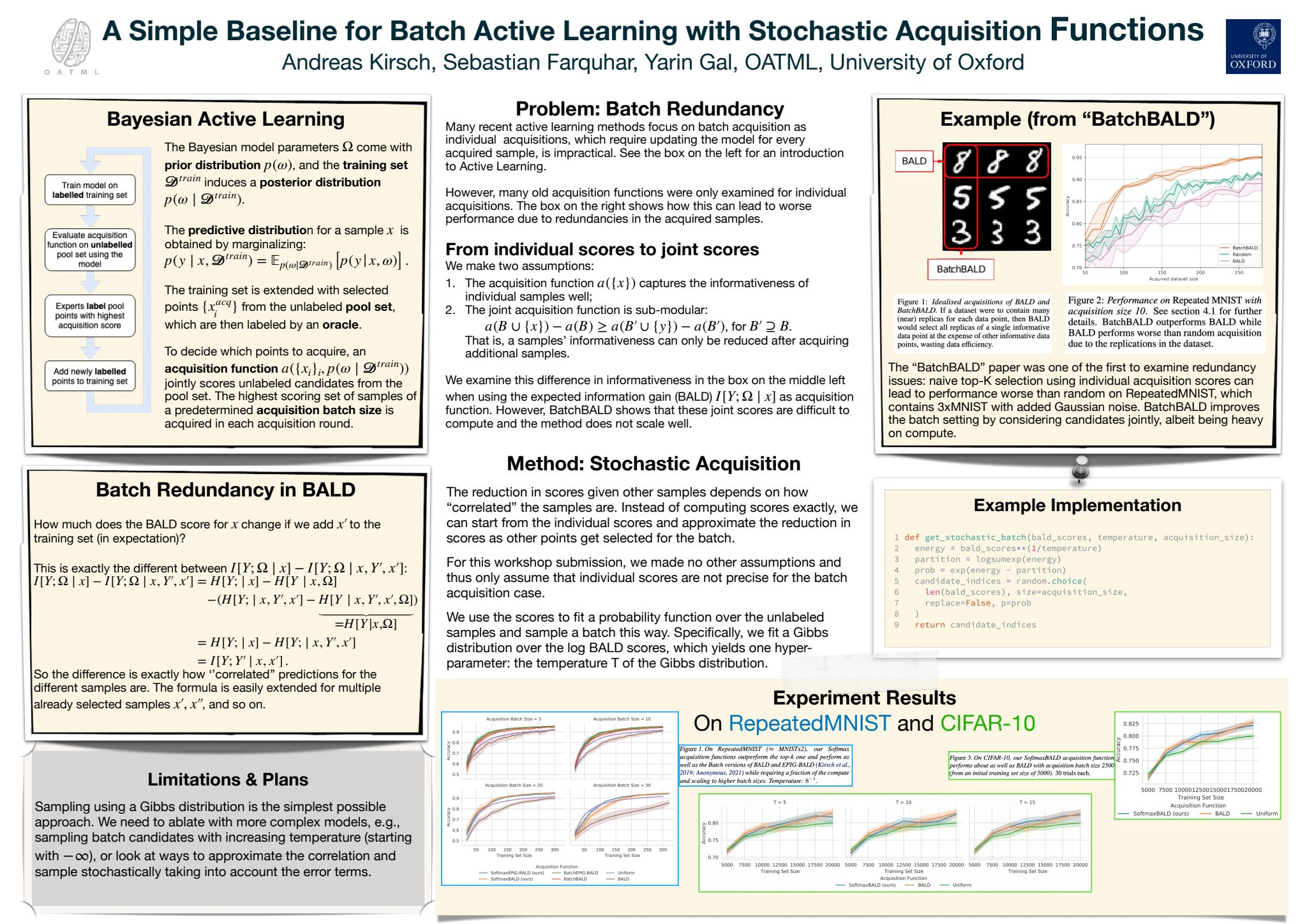
or as Poster PDF version as download.
A Practical & Unified Notation for Information-Theoretic Quantities in ML
Andreas Kirsch, Yarin Gal

or as Poster PDF version as download.
Prioritized training on points that are learnable, worth learning, and not yet learned
Sören Mindermann, Muhammed Razzak, Winnie Xu, Andreas Kirsch, Mrinank Sharma, Adrien Morisot, Aidan N. Gomez, Sebastian Farquhar, Jan Brauner, Yarin Gal
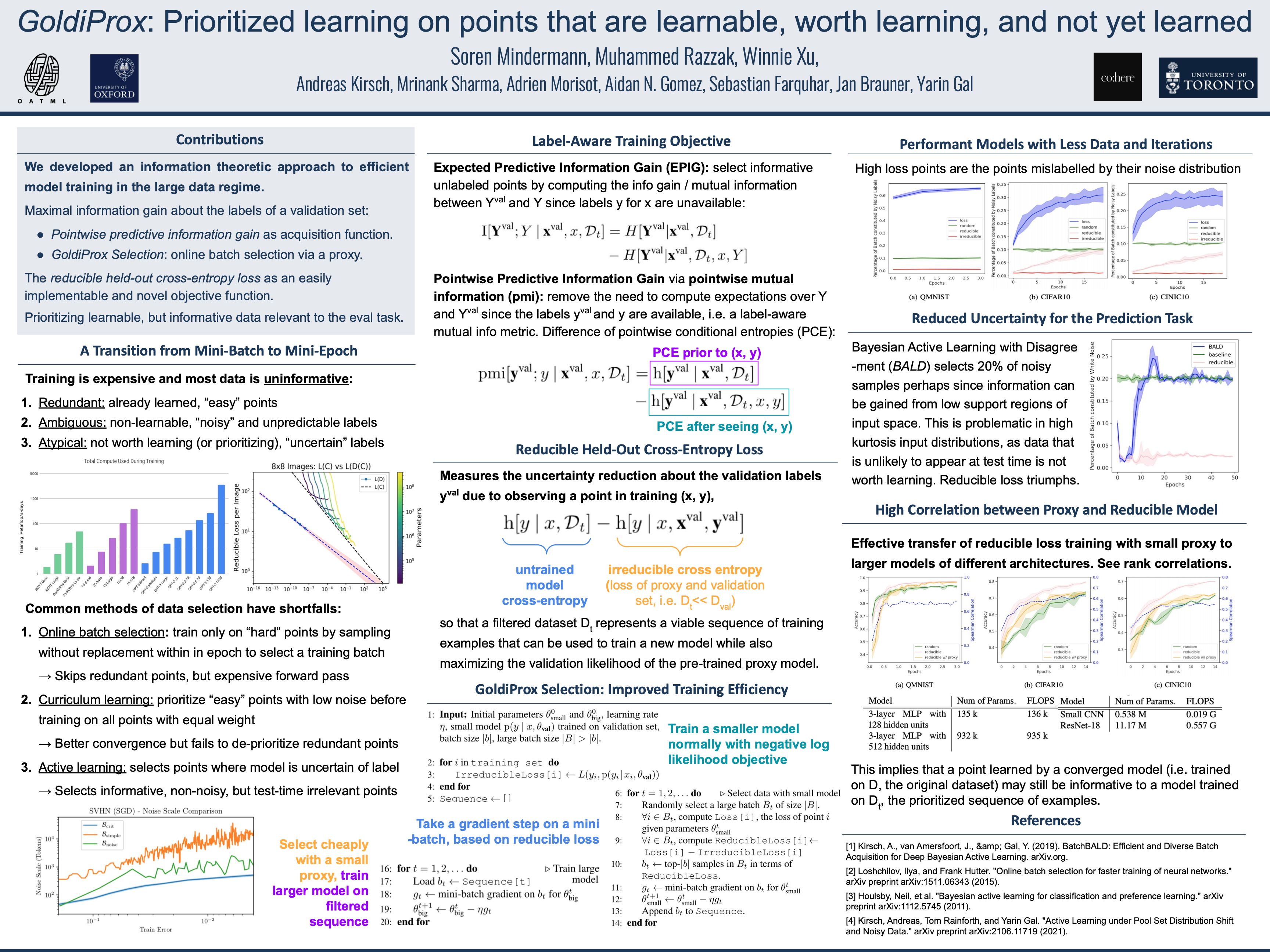
or as Poster PNG version as download.
One paper (poster) at the Neglected Assumptions In Causal Inference workshop:
|
Feb 23, 2021 |
Lecture on âBayesian Deep Learning, Information Theory and Active Learningâ for Oxford Global Exchanges. You can download the slides here.
|
Feb 21, 2021 |
Deterministic Neural Networks with Appropriate Inductive Biases Capture Epistemic and Aleatoric Uncertainty has been uploaded to arXiv as pre-print. Joint work with Jishnu Mukhoti, and together with Joost van Amersfoort, Philip H.S. Torr, Yarin Gal. We show that a single softmax neural net with minimal changes can beat the uncertainty predictions of Deep Ensembles and other more complex single-forward-pass uncertainty approaches.
|
Dec 10, 2020 |
Unpacking Information Bottlenecks: Unifying Information-Theoretic Objectives in Deep Learning was also presented as a poster at the âNeurIPS Europe meetup on Bayesian Deep Learningâ.
You can find the poster below (click to open):
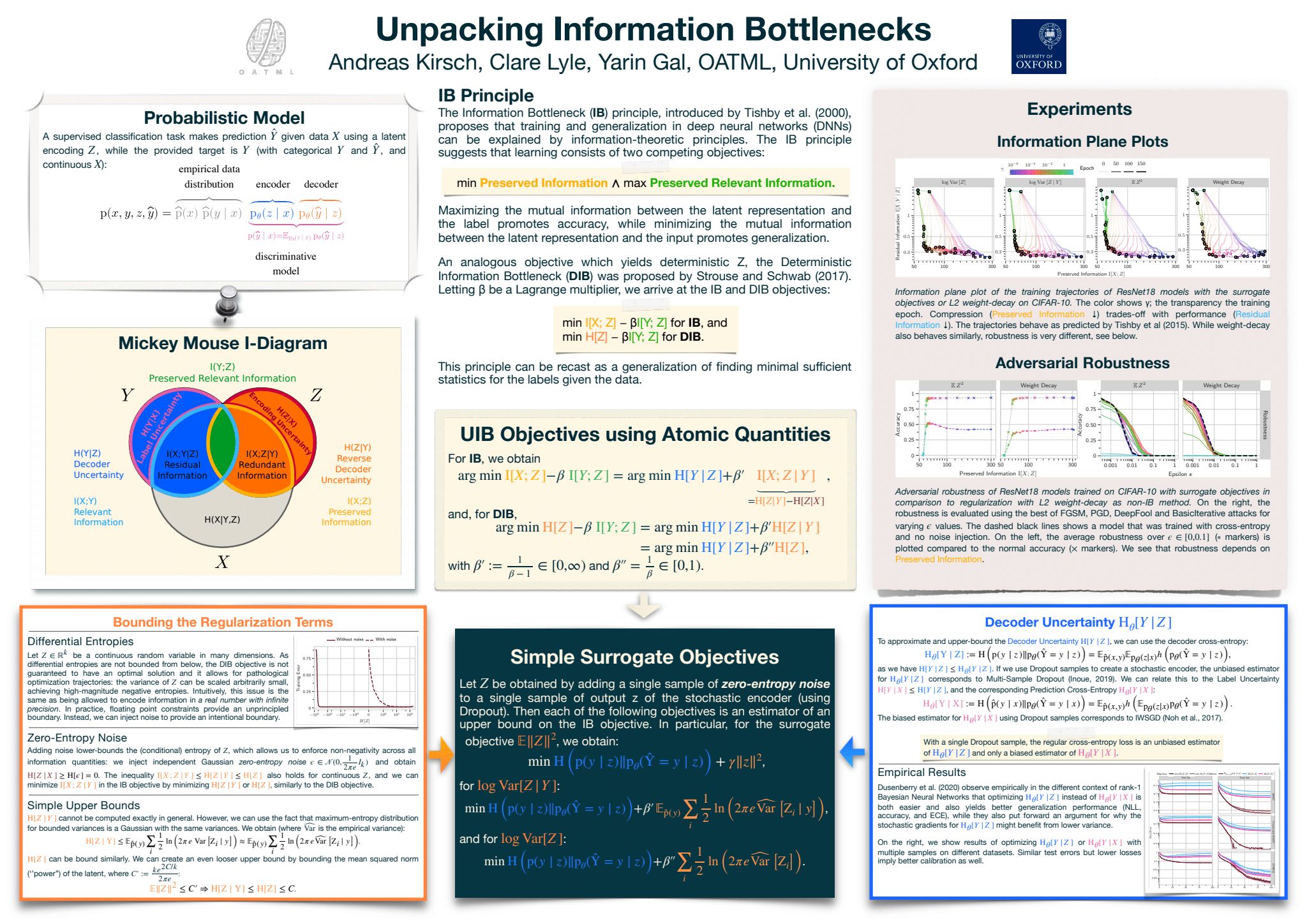
or as PDF version to download.
|
Jul 17, 2020 |
Two workshop papers have been accepted to Uncertainty & Robustness in Deep Learning Workshop at ICML 2020:
- Scalable Training with Information Bottleneck Objectives, and
- Learning CIFAR-10 with a Simple Entropy Estimator Using Information Bottleneck Objectives
both together with Clare Lyle and Yarin Gal. They are based on Unpacking Information Bottlenecks: Unifying Information-Theoretic Objectives in Deep Learning for the former, and an application of the UIB framework for the latter: we can use it to train models that perform well on CIFAR-10 without using a cross-entropy loss at all.
|
Mar 27, 2020 |
Unpacking Information Bottlenecks: Unifying Information-Theoretic Objectives in Deep Learning, together with Clare Lyle and Yarin Gal, has been uploaded as pre-print to arXiv. It examines and unifies different Information Bottleneck objectives and shows that we can introduce simple yet effective surrogate objectives without complex derivations.
|
Sep 4, 2019 |
BatchBALD: Efficient and Diverse Batch Acquisition for Deep Bayesian Active Learning got accepted into NeurIPS 2019. See you all in Vancouver!
|